18 min to read
R-Studio 주성분 분석 수행을 통한 데이터 상관 관계 분석 연습문제
R-Studio의 상관관계 분석 을 위한 procomp, eigen, factanal 함수 사용
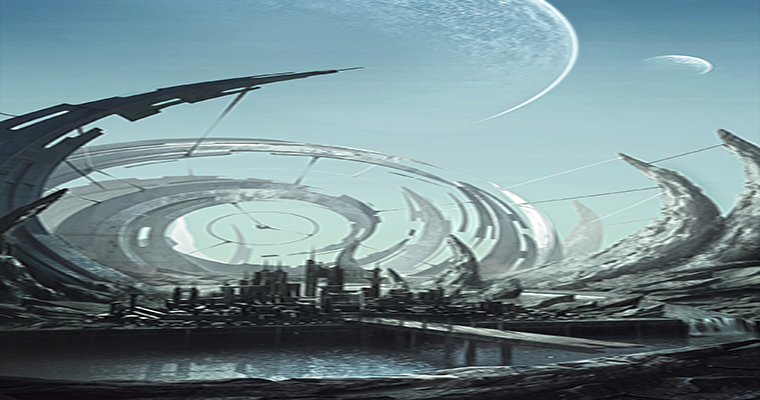
파일 소스
우클릭 -> 다른이름으로 링크저장 이용해 주세요
S20191104.zip
사용 함수
prcomp 주성분 분석 수행 함수
cor 상관계수 계산 함수
corrgeam 상관계수 도표 차트
factanal 요인 분석 함수
scatterplot3d 3d스캐터 차트 함수
사용 예시 소스코드 1
# 최소 제곱법(자승법)
test <- read.csv("linear_reg.csv", header = T)
head(test)
mean_x <- mean(test$x)
x <- test$x
mean_y <- mean(test$y)
y <- test$y
bunja <- sum((x-mean_x)*(y-mean_y))
bunja
bunmo <- sum((x-mean_x)**2)
a <- bunja/bunmo
b <- mean_y - a * mean_x
a
b
#
plot(test, pch='*', lwd=2, cex=2.0, col='blue')
abline(a=b, b=a, col="red", lwd=2)
# 머신러닝 학습 을 포뮬러 형태로 인자를 제공하고 나오는 결과물을 model 이라고 함.
# 그래서 변수명은 model로 정의.
model <- lm(y ~ x, data=test)
model
# Call:
# lm(formula = y ~ x, data = test) call 메소드
#
# Coefficients: 회기계수
# (Intercept) x 기울기 = x, 절편 = intercept
# 7.70 5.45
attributes(model)
# $names model이 가지고 있는 변수
# [1] "coefficients" "residuals" "effects" "rank" "fitted.values" "assign" "qr"
# [8] "df.residual" "xlevels" "call" "terms" "model"
#
# $class
# [1] "lm"
model$fitted.values
# y예측치(적합값)
# 1 2 3 4
# 149.4 160.3 171.2 182.1
head(test)
# x y
# 1 26 148
# 2 28 164
# 3 30 168
# 4 32 183
# head(test) - fitted.values : 잔차 라고한다.
model$residuals # 잔차 매개변수
summary(model)
# Call:
# lm(formula = y ~ x, data = test)
#
# Residuals:
# 1 2 3 4
# -1.4 3.7 -3.2 0.9
#
# Coefficients: 회기계수
# Estimate Std. Error t value Pr(>|t|)
# (Intercept) 7.700 23.764 0.324 0.7767
# x 5.450 0.817 6.671 0.0217 *
# ---
# Signif. codes: 0 ‘***’ 0.001 ‘**’ 0.01 ‘*’ 0.05 ‘.’ 0.1 ‘ ’ 1
#
# Residual standard error: 3.654 on 2 degrees of freedom
# Multiple R-squared: 0.957, Adjusted R-squared: 0.9355
# F-statistic: 44.5 on 1 and 2 DF, p-value: 0.02174
# x = 117이면 y=?
x <- c(110, 120, 130, 140, 150)
y <- c(100, 105, 128, 115, 142)
# 잔차의 제곱의 가장 이상적인 값은 0으로 가면 갈수록 좋다.
iq <- lm(y ~ x)
iq
plot(iq, pch='*', lwd=2, cex=2.0, col='blue')
사용 예시 소스코드 2
# df <- read.csv("test01.csv", header = T)
# df <- read.csv("test02.csv", header = T)
df <- read.csv("test03.csv", header = T)
df
x <- df$x
y <- df$y
model <- lm(y ~ x, data = df)
model
plot(df, pch='*', lwd=2, cex=2.0, col="blue", main="y=2*x+1 linear regression")
# coef : 회귀 직선 구하는 함수
abline(coef(model), col="red", lwd=2)
attributes(model)
model$coefficients # 회귀계수
model$fitted.values # 예측치
resi <- model$residuals # 잔차
resi_sqrt_total <- sum(resi**2) # 잔차 의 제곱의 총합
resi_sqrt_total
summary(model)
# Call: 포뮬러 정보.
# lm(formula = y ~ x, data = df)
#
# Residuals: 잔차
# 1 2 3 4
# 0.04 -0.12 0.12 -0.04
#
# Coefficients:
# Estimate Std. Error t value Pr(>|t|) p - value 값.
# (Intercept) 1.10000 0.15492 7.10 0.019263 *
# x 1.96000 0.05657 34.65 0.000832 ***
# ---
# # ***가 3개면 유의미하다.
# Signif. codes: 0 ‘***’ 0.001 ‘**’ 0.01 ‘*’ 0.05 ‘.’ 0.1 ‘ ’ 1
#
# Residual standard error: 0.1265 on 2 degrees of freedom
# R-squared : 상관계수
# Multiple R-squared: 0.9983, Adjusted R-squared: 0.9975
# F-statistic: 1201 on 1 and 2 DF, p-value: 0.0008319 # sqrt값이 이될수록 퍼펙트한 값이됨.
# 결정 계수가 1이면 일수록(상관계수) 유의미한 값이다. 반대로 가면 유의미 하지않은 값이다.(상관없는)
# p값이 0.05 보다 크면 부적합하다 라는 뜻이다.
sc <- read.csv("시험 점수와 공부 시간1.csv")
# sc <- read.csv("시험 점수와 공부 시간2.csv")
x <- sc$공부시간
y <- sc$학교성적
model <- lm(y ~ x, data = sc)
model
plot(x, y, pch='*', lwd=2, cex=2.0, col="blue", main="y=2*x+1 linear regression", ylim= c(0, 130))
# coef : 회귀 직선 구하는 함수
abline(coef(model), col="red", lwd=2)
attributes(model)
model$coefficients # 회귀계수
model$fitted.values # 예측치
resi <- model$residuals # 잔차
resi_sqrt_total <- sum(resi**2) # 잔차 의 제곱의 총합
resi_sqrt_total
summary(model)
2.463e-05 > 0.05 # FALSE
# 유의확률이 TRUE 면 회귀선이 모델에 부적합하다는 의미이다.
# 예시는 FALSE 이므로 적합하다고 볼 수 있다.
사용 예시 소스코드 3
# 파일 이름 : product.csv
# 제품의 적절성("appropriacy") 이 제품의 만족도("satisfaction") 에 얼마나 영향을 주는지
# 회귀 분석을 수행해보기
df01 <- read.csv("product.csv", header = T)
df01 <- df01[-1]
str(df01)
x <- df01$appropriacy # 독립 변수(종속 변수에 변화를 주는 변수)
y <- df01$satisfaction # 종속 변수(독립 변수에 의해 변화를 받는 변수)
model01 <- lm(y ~ x, data = df01)
model01
plot(df01, pch='*', lwd=2, cex=2.0, col="blue", main="y=2*x+1 linear regression")
# coef : 회귀 직선 구하는 함수
abline(coef(model01), col="red", lwd=2)
attributes(model01)
model01$coefficients # 회귀계수
model01$fitted.values # 예측치
resi <- model01$residuals # 잔차
resi_sqrt_total <- sum(resi**2) # 잔차 의 제곱의 총합
resi_sqrt_total
##########################################################################
# 파일 이름 : factor_analysis.csv
# 위의 파일을 이용하여 요인 분석을 수행하세요
fac_Analysis <- read.csv("factor_analysis.csv")
str(fac_Analysis)
# 상관 계수를 이용하여 개략적인 특징을 파악해 보세요.
pc <- prcomp(fac_Analysis)
summary(pc)
# Importance of components:
# PC1 PC2 PC3 PC4 PC5 PC6
# Standard deviation 2.9724 1.5844 1.4889 0.6763 0.44834 0.34049
# Proportion of Variance 0.6163 0.1751 0.1546 0.0319 0.01402 0.00809
# Cumulative Proportion 0.6163 0.7914 0.9460 0.9779 0.99191 1.00000
# 누적 비율을 보아 하니 PC3 까지의 누적 비율의 합이 94%가 넘어가므로 두개의 카테고리로
# 정리해야할 확률이 높다. 아직은 결정이 나지 않은 상태이다.
# 상관 계수를 그래프를 그려 보세요.
# plot 그리기
library(corrgram)
cor(fac_Analysis) # 상관계수 계산
corrgram(cor(fac_Analysis), upper.panel = panel.conf) # 상관계수 도표 그리기기
# 요인 분석은 기본적으로 상관 계수를 토대로 공통점을 인식한다.
# 주성분 요인수를 분석하세요.
# 요인수를 결정 하는 방법(values 이용)
en <- eigen(cor(fac_Analysis))
en$values # 고유치
# [1] 3.69391386 1.03419457 0.94752707 0.19286642 0.08178566 0.04971242
en$vectors
# [,1] [,2] [,3] [,4] [,5] [,6]
# [1,] 0.3927278 0.2606205 -0.54819213 0.5976675 -0.11344385 0.32749236
# [2,] 0.4196373 0.3310316 0.45128226 -0.3526018 -0.03339597 0.62066384
# [3,] 0.4144774 0.2890788 -0.46606690 -0.5635109 0.21591790 -0.40405262
# [4,] 0.4147989 -0.5545170 -0.04007911 -0.1554791 -0.69859479 -0.08147389
# [5,] 0.3972015 -0.6017487 0.05300804 0.1144664 0.67155730 0.11501251
# [6,] 0.4099400 0.2633209 0.52363648 0.4045301 -0.01925539 -0.56956190
# 주성분에 대하여 시각화를 수행해 보세요.
plot(en$values, type = 'o', pch = 19) # 엘보우 포인트 : 꺾은선이 완만하게 바뀌는 포인트
# 고유값을 보면 주성분을 3개로 간주 할 수 있다.
# p-value 구하기
# 어떤 데이터를 다른 데이터에 비해 부각 시키기 위해서 varimax 회전법을 많이쓴다 이는 디폴트 값이다.
# 요인을 분석하세요.
result <- factanal(fac_Analysis, factors = 3, rotation = "varimax", scores = "regression")
attributes(result)
# Loadings:
# Factor1 Factor2
# col1 0.362 0.385
# col2 0.974 0.216
# col3 0.496 0.385
# col4 0.243 0.967
# col5 0.244 0.879
# col6 0.874 0.245
# 요인 부하량 막대 그래프 표현
color <- rainbow(nrow(result$loadings[, c(1:3)]))
leName <- rownames(result$loadings[, c(1:3)])
barplot(result$loadings[, c(1:3)], beside = T, col = color)
legend(x = "topright", legend = leName, bty = 'o', fill = color)
# 각 요인별로 어느 변수가 많은 비율을 차지하는 지 시각화
library(plotrix)
threeLoad <- result$loadings[, c(1:3)]
first <- round(threeLoad[1:6]/sum(threeLoad[1:6])*100, 2)
second <- round(threeLoad[2:7]/sum(threeLoad[2:7])*100, 2)
third <- round(threeLoad[3:8]/sum(threeLoad[3:8])*100, 2)
# pie차트 그리기기
par(mfrow = c(1, 3))
firPie <- pie3D(x = first, main = "각 요인별 변수 차지 비율")
pec <- paste(rownames(result$loadings[, c(1:3)]), "\n", first, "%")
pie3D.labels(firPie, labels = pec, labelcex = 0.8)
secPie <- pie3D(x = second)
pec <- paste(rownames(result$loadings[, c(1:3)]), "\n", second, "%")
pie3D.labels(secPie, labels = pec, labelcex = 0.8)
thiPie <- pie3D(x = third)
pec <- paste(rownames(result$loadings[, c(1:3)]), "\n", third, "%")
pie3D.labels(thiPie, labels = pec, labelcex = 0.8)
# 요인 점수 및 시각화
# 3D scatter plot 그리기
# install.packages("scatterplot3d")
par(mfrow = c(1, 1))
library(scatterplot3d)
# 변수 맵핑
Factor1 <- result$scores[, 1]
Factor2 <- result$scores[, 2]
Factor3 <- result$scores[, 3]
loading1 <- result$loadings[, 1]
loading2 <- result$loadings[, 2]
loading3 <- result$loadings[, 3]
d3 <- scatterplot3d(Factor1, Factor2, Factor3, type = 'p', color='blue', angle = 40)
d3$points3d(loading1, loading2, loading3, bg="red", pch=21, cex=2, type = 'h')
# 요인별 산술 평균을 이용하여 변수의 갯수를 줄여 보세요.
# 요인별 산술 평균
f1 <- (fac_Analysis$col2 + fac_Analysis$col6)/2
f2 <- (fac_Analysis$col4 + fac_Analysis$col5)/2
f3 <- (fac_Analysis$col1 + fac_Analysis$col3)/2
f1
f2
f3
# 데이터 프레임 만들기
new_df <- data.frame(f1, f2, f3)
new_df
# 추려진 요인들을 이용하여 상관 관계 분석을 수행해 보세요.
library(corrgram)
cor(new_df) # 상관계수 계산
corrgram(cor(new_df), upper.panel = panel.conf) # 상관계수 도표 그리기기
Comments